Human-AI collaboration is becoming a workplace norm, but what do we know about optimizing it for humans? What types of collaborations decrease engagement?
Getting human-machine collaborations to succeed is not easy. While workers around the world are increasingly collaborating with smart machines, these machines have not yet been optimized for people, and people haven’t yet figured out how to maximize the value of these collaborations. Consider, for example, how Uber drivers, frustrated with the artificial intelligence (AI) that directs their driving, either identified clever ways around the algorithms, or manipulated them by artificially causing surge pricing.1 Or consider how call center turnover is often exasperated by AI solving simple customer problems, leaving call center workers to deal with the emotions of often the most unhappy and frustrated customers.2 Indeed, one study looking at those who closely collaborate with AI in the workplace revealed “persuasive evidence of negative effects on some dimensions of well-being.”3
Building successful human-machine collaborations will challenge most organizations to break down siloes between human resources and IT (information technology) and identify new strategies for improving human-machine teams. To engage and retain their talent, act responsibly to create positive experiences at work, and maximize the business potential of their tech investments, organizations should consider new approaches and strategies to encourage the success of human and machine collaborations.
We drew partly from extensive qualitative interviews in 30 companies where workers were collaborating with AI in the workplace to understand how AI and humans tend to collaborate, what challenges humans face when they collaborate, and how to foster positive relationships between humans and machines in the workforce.4
What Does “Human and Machine Collaboration” Really Mean?
In the summer of 2022, a board game designer named Jason Allen made national headlines for winning a minor prize in the Colorado State Fair’s art competition. While his painting, titled Théâtre D’opéra Spatial, was beautiful—at least in the eyes of the judges—he became a national sensation for the method by which he produced his winning painting: He developed the piece of art using a generative AI tool that turns plain language queries into unique images. Not surprisingly, artists and critics immediately expressed outrage that the painting represented “the death of artistry”5 and that his effort represented “the literal definition of ‘pressed a few buttons to make a digital art piece.’”6
But the process wasn’t so simple. By his own estimate, Allen spent approximately 80 hours testing and improving different textual prompts in the AI tool, tweaking the image output digitally, and enlisting multiple digital tools to create what might best be thought of as a collaborative painting effort between a human and a machine. Allen describes himself as “the author” of the painting rather than a painter or artist.7
While it might be tempting to dismiss Théâtre D’opéra Spatial as a stunt, it represents the kind of collaborative approach that is expected to increasingly shape how humans work with machines—in not just the arts but across the world of work. Allen isn’t the only such “author”—there is at least one startup that serves as a marketplace for “prompt engineers” to sell tailored prompts for generative art and writing tools.8 Such collaborations are raising questions about identity, allocation of rewards when work is done jointly by humans and AI, and the transformation of the workforce experience when work is done not just by people but by people in collaboration with AI.
Over the last decade, we’ve seen an explosion of AI tools aimed at empowering workers by automating routine work to let human workers focus on more complex, nuanced tasks. This is only a narrow form of human and machine collaboration. A potentially more valuable form of human and machine collaboration involves workers actively interacting with AI consistently during their regular workday—requiring them to not just “friend” and collaborate with their human colleagues, but to “friend” and collaborate with smart machines too.
There are many types of daily interactions workers can have with AI (figure 1), ranging from people working with AI to supervise AI’s work (machines as subordinates), to people working with AI in a way that directs their work (machines as supervisors), to people working with AI in open-ended, highly iterative, and interactive ways over time in true partnership (machines as teammates).
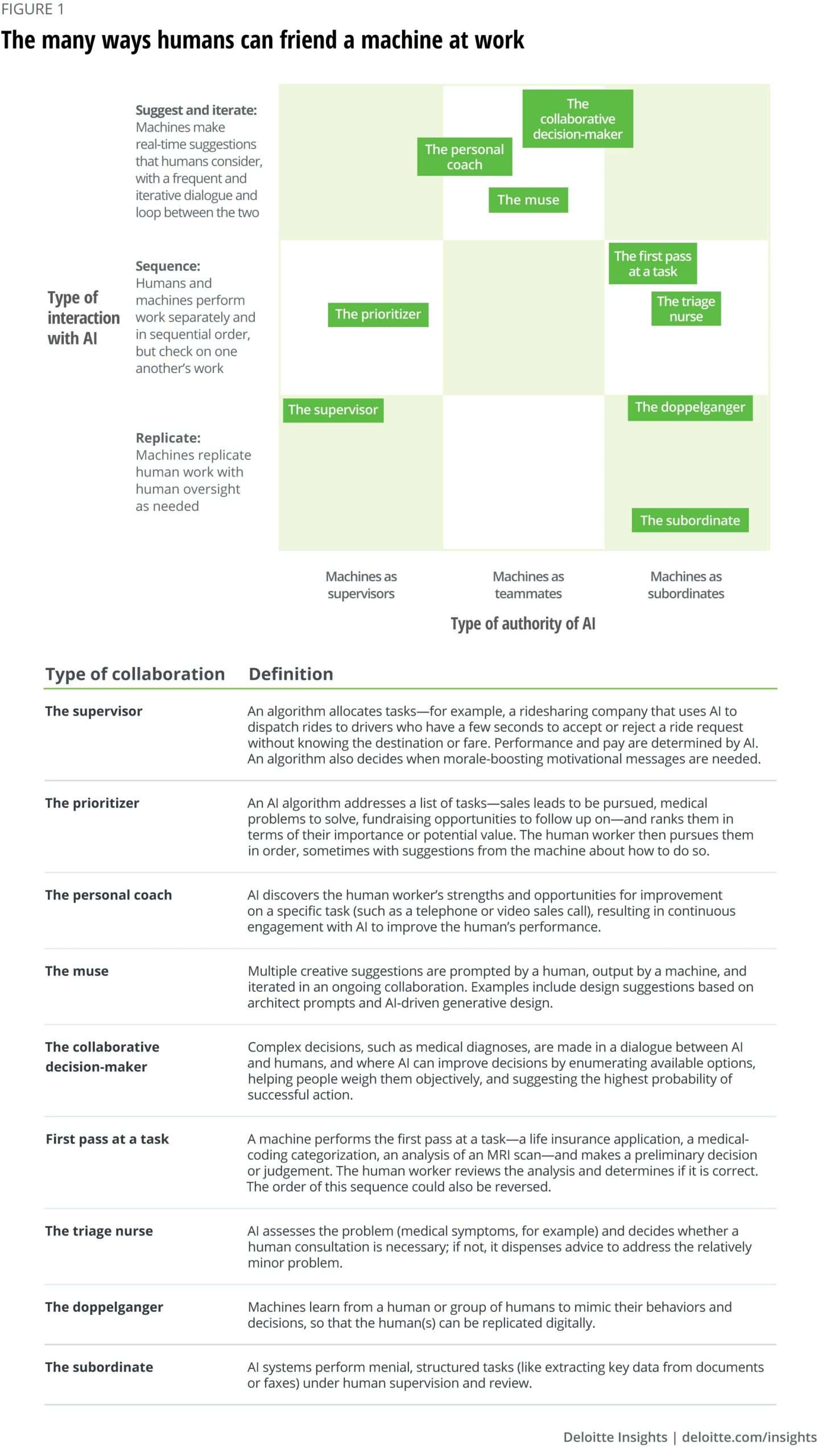
While interacting with machines as subordinates or supervisors is increasingly common (think warehouse workers and rideshare drivers, for example, whose jobs involve largely taking tasks and directions from AI systems), interacting with machines as teammates is only still emerging. Like Jason Allen using an AI tool as a muse to create a novel piece of art, we expect to increasingly see teams of human-machine collaborators working together in open-ended, highly iterative, and interactive ways over time. These kinds of collaborative AI tools are emerging in a wide variety of fields, ranging from agriculture to medical diagnostics to software development. They are taking on increasingly complex, higher-stakes roles working with people to perform work—from prioritizing key tasks to shifting the locus of decision-making within an organization.
Large language models, like image generation AI systems, are increasingly powering human-machine collaborations involving prompts from humans interacting with AI in the workplace. Systems such as OpenAI’s GPT-3 and Google’s LaMDA can create articles, conversations, blog posts, product descriptions, and multiple other content types in response to human prompts. They can produce programming code as well acting as a kind of coding muse—in multiple languages. One engineer emphasized how different collaborating with AI is from collaborating with human colleagues: “It’s a very foreign intelligence, right? It’s not something you’re used to. It’s not like a human theory of mind. It’s like an alien artifact that came out of this massive optimization.”9
This idea—that humans need to develop a “theory of mind” of AI collaborators—extends to other areas such as airline safety, where aviation-safety experts argue that pilots need more training to fly planes with collaborative autopilot systems because they “must have a mental model of both the aircraft and its primary systems, as well as how the flight automation works” to manage issues that could turn into catastrophic crashes.10 This is because collaborative AI tools simultaneously increase productivity but also create new and different kinds of vulnerabilities and potential problems. For example, one study of 1,689 software programs generated by working with code-generation tools found that 40 percent had security flaws.11 This potential for vulnerability not only raises concerns for cybersecurity professionals but also for HR professionals who will need to organize the output of human-machine partnerships. To ensure that these collaborations succeed, it will be critical to make sure that the human workforce is prepared to learn new ways to collaborate with digital coworkers.
How Human and Machine Collaboration Challenges the Workforce Experience
Human and machine collaboration can significantly alter the workforce experience based on the type of interaction with AI—sometimes for the worse. Organizations are developing innovative responses to three major challenges workers face when interacting with AI in the workplace.
AI Makes Work Harder for Humans
AI sometimes increases the level of difficulty and complexity of the work since AI systems often handle the easier tasks, asking input from the humans on the more complex ones. This is especially true when AI acts as a prioritizer, triage nurse, subordinate, or performs a first pass at a task. In medical coding, for example, AI-based systems taking the initial pass at coding a case from electronic records and notes means that companies may need only experienced coders who can audit the system’s decisions—not entry-level ones.12 And many experienced doctors now work more with AI rather than junior professionals who could learn from them.13 As Matt Beane points out in his Harvard Business Review article, “Learning to work with intelligent machines,” this can result in increasingly fewer entry-level roles and opportunities to learn from others.14
To address this issue, the primary focus, if any, has been upskilling or reskilling people to take on harder work demanding new skills, including arming workers with “digital or AI fluency” to be able to interact with AI. While “reskilling” or “upskilling” has been the primary focus of many organizations’ people-oriented AI initiatives, research suggests organizations are still underinvesting: In a recent Deloitte survey, only 5% of leaders strongly agreed that they’re investing enough in helping people learn new skills to keep up with changes like digital transformation, though 73% said they’re accelerating upskilling or reskilling efforts.15
Some jobs are being redesigned to reflect increased responsibilities and to ensure that workers are hired, developed, rewarded, recognized, and compensated accordingly for the more difficult, and often far more valuable, work. Actuaries, for example, are increasingly working with AI to predict and identify risk. Actuary professional associations now require training on core machine learning and data science skills, in part because the distinction between an actuary and a data scientist is gradually disappearing. And as AI helps actuaries with the assessment of risk, many organizations are now redesigning job descriptions and hiring and rewarding actuaries based on nontechnical skills like judgment, decision-making, adaptability, and change management.16
For those collaborations in which AI acts a teammate, collaborations will likely be a mix of more complex work and easier interactions. On the surface, AI makes work easier—suggesting and coauthoring code with human engineers, weighing in on a medical diagnosis, or giving salespeople immediate feedback on how to improve interactions with customers.
But in many ways, working with AI as a teammate can be much more demanding. Human workers may need to understand and reflect on how AI does its work and assess whether its conclusions and outputs are correct. Training humans to collaborate with machines may demand a set of skills that go far beyond “digital fluency” and reskilling. Organizations may need to pair AI system and process designers with HR professionals to collaborate on how to support these new types of interactions.
Organizations should also look for ways to move some human-machine actions toward AI serving as a coach. This can create a continuous cycle of reinforcing and supporting increasingly more difficult work. Hewlett Packard used AI, for example, to listen to and learn from customer calls to determine each call center agent’s unique “microskills”—the agent’s knowledge of a specific kind of customer request—captured from previous calls. These microskills are now used to match incoming calls to agents who have successfully processed similar requests. As customer service agents learn new skills, the AI software automatically updates its data to reflect their new expertise. As these agents become more knowledgeable, the software learns to route more complex problems to them. As the call center agent engages in calls, the software continually learns from the agent, enabling the software to embed agent knowledge and expertise into its algorithms.17
AI-Structured Work May Increase Short-Term Productivity but Can Decrease Autonomy and Engagement
While subordinate forms of AI often create more complex work for humans, supervisory AI can push workers into rigid structures and processes that can reduce autonomy and ultimately impact performance over time. Working for an AI supervisor can limit or reduce decision-making power and increase employee monitoring. We have found examples of both limited decision power and increased monitoring in insurance underwriting, medical coding, mortgage processing, clothing-style selection, call center work, and policing.
Some workers, of course, enjoy having a more structured work experience with the help of AI (though many will not). Many research studies have found that most people are quite happy to be directed and managed by AI,18 and that they often trust AI more than their managers.19 However, research reveals that many start to feel differently when a worker disagrees with AI or when they have new ideas or want to influence changes to their work that AI can’t accommodate, or if AI structures their work in a way that only maximizes business results without considering workers’ needs as well.20 Employee aptitudes and work-style preferences should be considered when filling a role.
Workers should also be involved in designing AI systems. Imagine if an algorithm directing drivers’ routes for maximum efficiency could also accommodate their scheduling preferences. Higher levels of motivation might compensate for a slight compromise in efficiency. However, today only 30% of leaders surveyed report including workers in participative design of AI.21 In addition, explainable AI (explainable to workers, not just to developers or leaders) can help make clear the underlying logic of the AI, building trust. All too often, while AI is learning a lot about them, workers know little about the AI. Although it may not be possible or even useful to share the algorithm itself with workers, leaders can and should share with them the data and goals that informed it.
In some cases, workers may design the AI or automation workflows entirely by themselves using low-code or no-code tools—giving them the autonomy to design the system that they may interact with as subordinates or supervisors to meet their needs. At the software company Tonkean, for example, operations teams were empowered to use such tools to build custom workflows without needing to rely on developers. They were also able to take a people-first approach. Instead of focusing process design primarily on business needs before thinking about the impact to employees, teams created processes that took their own needs into account as well.22
Organizations should also incorporate worker feedback loops. Workers should be encouraged to question an algorithm and even challenge or override it. They will also need ways to report problems or unfair treatment. Ideally, the technology itself would have a built-in feedback loop to help the algorithm learn and improve over time.
AI can also act as a coach to give workers greater empowerment to make decisions instead of those decisions being made higher up, or by algorithms themselves. AI can observe people’s decision-making effectiveness and make recommendations. Klick Health’s machine-learning technology, “Genome,” is designed to help employers manage autonomy. By analyzing every project at every stage in the firm, it rewards more responsibility to people who have demonstrated consistent competency and success. The AI tracks every decision made in the firm and the context in which it was made.23
AI itself can even help mediate the relationship between AI and workers, giving workers more choice and autonomy when working with AI. Consider the AI-powered tool developed by Vecna Robotics, named Pivotal, which allows warehouse workers and robots with different skills and abilities to bid on what they feel they can or want to do. Instead of doing the same shelf-picking tasks all day long, with Pivotal, workers often end up finding variety in their jobs, handling unusual tasks such as clearing obstacles and applying their abstract problem-solving skills to understanding why a box had two conflicting labels and deciding which one was right. Robots tend to settle into repeatable patterns with gradual performance improvement until their engineers analyze the data and push out new features that enable broader skillsets. Machine learning benefits from human insights and human training of AI algorithms, and humans analyze the unexpected behaviors and emerging patterns and innovate—both on the shop floor and in the engineering office. The symbiotic human-AI system was designed to emphasize autonomy, responsibility, competence, and diversity of intelligence.24
Collaborating with AI Can Increase Loneliness, Isolation, and Questions of Identity
A common theme we heard from those interviewed is that working collaboratively with AI can make human workers lonely and isolated, thereby suggesting that organizations may need to consciously foster social interactions. Many collaborative AI systems provide all the data and insights employees need to do their jobs, and they often have less interaction with other humans as a result. A group of “digital life underwriters” of insurance, for example, only have an AI system as their daily companions.25 In one hospital, an intelligent robot that fills prescriptions and a pneumatic tube distribution system for those prescriptions meant that pharmacists no longer had to deliver medications to patient floors and were relegated to basement cubicles for phone consultations with physicians.
Recognizing the potential for people interacting with AI frequently to isolate them and reduce engagement, many employers have responded by intentionally building social interaction back into their work lives. The digital life underwriters mentioned above, for example, meet weekly (virtually) with other underwriters and AI system developers to discuss improvements in the system and underwriting process. Uber now offers in-app and phone support for drivers who have a question or need help.26 Organizations should retain human managerial and assistance roles and help people foster connections with their coworkers, like facilitating human-to-human interactions through affinity groups, social events, and online communities.
For organizations looking to integrate collaborative AI as a teammate, it will be important to develop systems that do more than improve performance on a specific task. They should also contextualize performance within broader team dynamics. They can also use AI to facilitate more or better-quality social interactions.27 Researchers in Norway, for example, have created an AI system that recommends new organizational structures to facilitate communications among workers who need to collaborate with each other.28
Interacting frequently with AI can also raise questions of identity. When AI is acting as a supervisor, for example, it can leave some workers feeling like they’re in turn treated like a machine—following the directions of the algorithm based on numbers for the sake of efficiency without much room for humanity. And when people work with AI as a kind of digital doppelganger of themselves—with AI analyzing a person’s digital footprint to mimic their behaviors and decisions so that the human(s) can be replicated digitally—the question of identity can become particularly acute.
Already, we see foreshadowing of what could happen in the workplace in the entertainment industry; a company claimed the rights to use Bruce Willis’ AI-based “deep fake” persona, for example, only for his representatives to deny they had sold the rights to his “digital doppelganger.”29 AI-powered replicas of real people are now taking on the jobs of entertainers, law enforcement, and more.30 This may raise questions in the future like: Who owns the intellectual property? If AI is used to learn from or mimic me, is part of “me” now embedded in the AI? What happens if the AI doesn’t accurately represent “me” or my knowledge as I want it to?
Tech companies are just starting to develop capabilities to create a kind of digital clone of people at work: Microsoft patented a chatbot that can act like real people based on their social media profile,31 and a team at MIT Media Lab is working on technology to enable machine intelligence to replicate a person’s digital identity so that others can “borrow their identity” to provide consultation or to help with decision-making in the absence of the source human.32
Although these examples may seem far-fetched, a growing number of companies are starting to enter the next phase of evolution of human and machine collaboration—moving from machines learning by processing vast amounts of data to machines being trained by human experts, in which the skills, capabilities, and knowledge of everyday workers are embedded into the algorithms themselves. Explains Gurdeep Pall, Microsoft’s corporate vice president of business AI, “Machine learning is all about algorithmically finding patterns in the data. Machine teaching is about the transfer of knowledge from the human expert to the machine learning system.”33
Regarding questions of identity, organizations may need to provide rewards and incentives to the human specialists whose knowledge is now embedded in AI—rewarding those specialists for the work that is now performed by AI but that is based on the sharing of their personal knowledge and experience. When machines act as true teammates, organizations should establish clear guidelines for establishing credit and accountability for joint work. When a jointly completed life insurance application results in an underwriting loss, for example, who gets a bad performance review—the human or the AI-based underwriter?
General Principles to Encourage the Success of Human and Machine Collaborations
Fostering successful human and machine collaboration should include paying close attention to how it can transform the workforce experience, with specific strategies put in place based on the type of human and machine interactions. But there are also some general principles that apply to improving all forms of AI and human collaboration—and that go far beyond traditional change management, reskilling, or responsible AI where most organizations stop.
Appoint Someone to Study and Optimize Human-Machine Collaboration
Until recently, many organizations have not paid close attention to how working with AI impacts the worker experience, and how to optimize the relationships of AI and humans for the good of both workers and the organization. But leading organizations are increasingly paying attention to this—Forty-three percent of global executives surveyed in Deloitte’s recent State of AI research report that their organization has appointed a leader responsible for helping humans collaborate better with intelligent machines.34
It’s not clear who this leader is, however. It may be a chief analytics or AI, information, data, digital, or technology officer, but rarely are chief human resources officers involved in human-machine collaboration design. They may be involved in issues related to culture change, lost jobs due to automation, reskilling, helping workers gain digital fluency, or the use of AI in talent and HR practices specifically within the HR function. But optimizing the human and machine relationship requires multidisciplinary expertise from both tech and people domains.
It may even be time to make the CHRO the CHRMO—or chief human+machine resource officer—responsible for a workforce that is now composed of workers working collaboratively with AI, and where the line between technology and people is increasingly blurred. Explains Jacqui Canney, chief people officer at ServiceNow and former executive vice president of the Global People division for Walmart Inc., “In an augmented workforce, the traditional boundary between humans and machines disappears. This will require CHROs to take on a new and significantly expanded role of managing the joint performance of humans working more closely with smart machines.”35 According to one research study, nearly 80 percent of C-suite executives surveyed believe the CHRO role should oversee not just HR, but HMR (human and machine resources).36
No matter who takes on the role, leaders should observe and study how AI and human collaborations work effectively—using techniques of anthropological research, surveys, or sentiment analysis, or even using sophisticated new technologies to collect data that can be analyzed on the effectiveness of human and machine collaboration.
Redefine Workforce Practices with Human and Machine Collaboration in Mind
No matter who takes the lead on optimizing human and machine collaboration, HR will likely need to reinvent its workforce practices in light of workers increasingly collaborating with smart machines. This is still a nascent and developing area—only 36% of large global companies surveyed report redesigning talent practices for a mixed human and machine workforce.37 We have not observed many adopting such practices.
Compensation and rewards, for example, could be adjusted for new AI and human collaborations. Organizations could make employees’ performance reviews—and their subsequent bonuses and salaries—commensurate with how well they work with machines. Performance appraisals based on joint human and machine performance could help workers share in the wealth that AI creates—especially if it’s AI that has learned from the workers themselves. Eighty-six percent of workers surveyed who work with smart machines believe their pay, performance ratings, and feedback should be based on their joint human-machine performance.38
Human and machine collaboration also raises the issue of how to define and use workforce performance measures, as what work inputs and outcomes mean changes when work is done collaboratively with smart machines. Measures like revenue per employee, for example, may already be obsolete. If AI is now in part responsible for the work that humans produce to contribute to financial success, then this metric provides only a partial, and perhaps misleading, view. The same is true for cost metrics—should we measure labor cost, or is cost of work a more relevant metric—reflecting the cost regardless of who in combination performs the work—whether it be employees, workers in collaboration with AI, or even external contributors?39
Careful thought and design of AI and human collaboration will also be needed for humans and machines to mutually communicate their capabilities, goals, and intentions—with HR stepping in to help workers clearly define their roles (and identities) as they work with AI. And as they plot how machines and humans will collaborate, HR professionals may need to go beyond redefining the roles of workers and the skills and capabilities needed to perform them. They may also need to shift from “organization design” to “work design” with a variety of resources that come together (humans and machines, employees and external contributors) and work synergistically. In workforce planning, leaders may need to learn how to look underneath the job at the activity and skill level to make choices about not only what to automate, but what type of work will need what type of human and machine collaboration—ranging from machines as subordinates, to supervisors, to teammates. When deploying workers into various roles, HR leaders could also carefully match the type of AI collaboration to the type of worker based on their unique work styles and preferences.
Finally, the physical workplace should be considered in light of emerging human and machine collaborations—in particular, teams of people working with AI as a teammate. There are design firms that can help organizations design office spaces that involve human-machine collaboration.40 AI is also already being used to help design office environments that fit employee desires for hybrid work.
Prepare Now for an Evolving Workforce
AI may be a powerful technology, but nothing will get better by simply adding AI to existing workflows or tasks, or by treating AI separately from workers. Instead, AI needs to be woven into collaborative processes with people—with careful attention paid to optimizing the experience of workers interacting with it. But there are many forms that human-machine collaboration can take—understanding the varying types and how they impact the experience of workers in different ways is important to optimizing this new relationship.
Increasingly, we see that the more commonly used interactions with machines as supervisors or subordinates are evolving into machines as teammates. HP’s call center AI system, for example, evolved from AI acting as a supervisor (routing customer calls to the agents with the skills best equipped to handle them), to AI acting as a digital doppelganger (learning the skills and expertise of agents to embed it into its algorithms), to eventually, AI also acting as a coach and teammate, suggesting to agents in real time how to handle calls based on the collective wisdom of the agents.41
These “machines as teammates” collaborations involve ongoing interactions between human workers and AI systems that need to work together in complex and changing ways to achieve a goal. Ensuring that these collaborations are successful, however, will challenge leaders to blend disparate fields such as HR and interaction design and develop new processes and approaches to structure these collaborations.
The technology that enables humans and machines to collaborate is ready for business. The question is: Is your business ready to unleash the potential of human and machine collaboration to not only drive new opportunity for the business, but also for your workers and society at large?
In recent years, many have talked about treating AI as a kind of “digital worker,” anthropomorphizing it and applying the same workforce management practices to AI as we do to employees. In this view of the world, there is a kind of “Artificial Intelligence Life Cycle” akin to the “Employee Life Cycle.” AI is considered a talent source when hiring—it needs to be “recruited, selected, and onboarded”—and is given a performance review, set of key performance indicators (KPIs), place in the organization chart, training and development, and a retirement plan. Organizations like NASA already give AI systems employee IDs, enabling them to better integrate with IT systems.42 Gartner even predicts that HR departments in the future won’t focus only on human employees, but also include a “robot resources” department to look after nonhuman workers. By 2025, Gartner predicts, at least two of the top 10 global retailers will have reshuffled their HR departments to accommodate the needs of their new robot workers.43
But should we consider AI as a kind of digital employee with the ability to learn, grow within the company, and interact as a member of the team—just like a human employee? While this may make sense for AI as automation—where a machine is working on its own—it doesn’t make as much sense in a world where workers are increasingly collaborating with AI, often in ways that make it hard to distinguish where the AI work begins and the human work ends. Anthropomorphizing AI is a mindset that assumes work happens sequentially, in siloes, and independently. In short, treating AI as distinct actors from humans doesn’t get at the heart of helping workers collaborate effectively with AI—which is where real performance improvements can be achieved.
- Sam Sweeney, “Uber, Lyft drivers manipulate fares at Reagan National causing artificial surge prices,” WJLA News, May 16, 2019. View in Article
- Francois Candelon, Su Min Ha, and Colleen McDonald, “A.I. could make your company more productive—but not if it makes your people less happy,” Fortune, January 7, 2022. View in Article
- Luísa Nazareno and Daniel S. Schiff, “The impact of automation and artificial intelligence on worker well-being,” Technology in Society 67, November 2021. View in Article
- The case studies and lessons learned from them are found in Thomas H. Davenport and Steven Miller, Working with AI: Real Stories of Human-Machine Collaboration (MIT Press, 2022). View in Article
- Matthew Gault, “An AI-generated artwork won first place at a state fair fine arts competition, and artists are pissed,” Vice, August 31, 2022. View in Article
- Rachel Metz, “AI won an art contest, and artists are furious,” CNN Business, September 3, 2022. View in Article
- Anna Lynn Winfrey, “After AI painting wins at Colorado State Fair, Pueblo artist explains,” The Pueblo Chieftain, September 1, 2022. View in Article
- Adi Robertson, “Professional AI whisperers have launched a marketplace for DALL-E prompts,” The Verge, September 2, 2022. View in Article
- Clive Thompson, “Copilot Is like GPT-3 but for code—fun, fast, and full of flaws,” WIRED, March 15, 2022. View in Article
- Shelm Malmquist and Roger Rapoport, “The plane paradox: more automation should mean more training,” WIRED, April 24, 2021. View in Article
- NYU Tandon School of Engineering, “Award-winning Tandon researchers are exposing the flaws underwriting AI-generated code,” June 16, 2022; Hammond Pearce et al., “Asleep at the keyboard? Assessing the security of GitHub Copilot’s code contributions,” Cornell University, December 16, 2021. View in Article
- Davenport and Miller, Working with AI. View in Article
- Matt Beane, “Learning to work with intelligent machines,” Harvard Business Review, September–October 2019. View in Article
- Ibid. View in Article
- Sue Cantrell et al., The skills-based organization: A new operating model for work and the workforce, Deloitte Insights, September 8, 2022. View in Article
- Andrew Hetherington, “New actuaries must know about machine learning,” Towards Data Science, May 30, 2020; McKinsey, “Reimagining actuaries: A Q&A with Society of Actuaries’ Greg Heidrich,” interview, 2020. View in Article
- Paul R. Daugherty and H. James Wilson, “Using AI to make knowledge workers more effective,” Harvard Business Review, April 19, 2019; Simon Firth, “HP labs uses artificial intelligence to improve HP’s customer service offerings,” Hewlett Packard Labs, January 15, 2020. View in Article
- Carnegie Mellon study referenced by: Adi Gaskell, “How we respond to being judged by algorithms at work,” Forbes, September 14, 2021. View in Article
- Dave Zielinski, “Why employees trust robots more than their managers,” SHRM, December 3, 2019. View in Article
- Adi Gaskell, “How Uber drivers feel about being managed by machines,” Forbes, May 8, 2018; Ning F. Ma et al., Using stakeholder theory to examine drivers’ stake in Uber, CHI 2018: Proceedings of the 2018 CHI Conference on Human Factors in Computing Systems, April 2018; Mareike Möhlmann and Lior Zalmanson, Hands on the wheel: Navigating algorithmic management and Uber drivers’ autonomy, Conference: International Conference on Information Systems (ICIS 2017), December 2017. View in Article
- Nitin Mittal, Beena Ammanath, and Irfan Saif, “State of AI in the Enterprise, 5th edition,” Deloitte, October 2022. View in Article
- Terri Griffiths, “How-to: Work crafting with automation,” accessed June 20, 2022. View in Article
- Allan Schweyer, “The impact and potential of artificial intelligence in incentives, rewards, and recognition,” Incentive Research Foundation, 2018. View in Article
- John Paschkewitz and Dan Patt, “Can AI make your job more interesting?,” Issues in Science and Technology 37, no. 1 (2020). View in Article
- Thomas H. Davenport, “The future of work now: The digital life underwriter,” Forbes, October. 18. 2019. View in Article
- Dr. Mark van Rijmenam, “Algorithmic management: What is it (and what’s next)?,” Medium, November 13, 2020. View in Article
- Monika Mahto et al., AI for work relationships may be a great untapped opportunity, Deloitte Insights, October 24, 2022. View in Article
- Nicolay Worren, Tore Christiansen, and Kim Verner Soldal, “Using an algorithmic approach for grouping roles and sub-units,” Journal of Organization Design 9 (2020). View in Article
- Ben Derico and James Clayton, “Bruce Willis denies selling rights to his face,” BBC News, October 1, 2022. View in Article
- Anthony Green, “How AI is helping birth digital humans that look and sound just like us,” MIT Technology Review, September 29, 2022. View in Article
- Grace Kay, “Microsoft has patented a chatbot that could imitate a deceased loved one, celebrity, or fictional character,” Business Insider, January 22, 2022; Dalvin Brown, “Microsoft patent would reincarnate dead relatives as chatbots,” Washington Post, February 4, 2021. View in Article
- MIT Media Lab, “Augmented eternity and swappable identities,” accessed October 17, 2022. View in Article
- Jared Newman, “Microsoft wants machine teaching to be the next big AI trend,” Fast Company, April 23, 2019. View in Article
- Mittal, Ammanath, and Saif, “State of AI in the enterprise, 5th edition.” View in Article
- Ellyn Shook, Mark Knickrehm, and Eva Sage-Gavin, Putting trust to work, Accenture, accessed November 14, 2022. View in Article
- Ibid. View in Article
- Mittal, Ammanath, and Saif, “State of AI in the enterprise, 5th edition.” View in Article
- Shook, Knickrehm, and Sage-Gavin, Putting trust to work. View in Article
- For a discussion of such metrics, see: “Human capital as an asset: An accounting framework to reset the value of talent in the new world of work,” World Economic Forum in collaboration with Willis Towers Watson, August, 2020. View in Article
- Scott Berinato, “What is an office for?,” Harvard Business Review, July 15, 2020. View in Article
- Daugherty and Wilson, “Using AI to make knowledge workers more effective,” April 19, 2019; Firth, “HP labs uses artificial intelligence to improve HP’s customer service offerings.” View in Article
- Craig Le Clair, “RPA operating models should be light and federated,” Forrester, August 18, 2017. View in Article
- Daphne Leprince-Ringuet, “The growing robot workforce means we’ll need a robot HR department, too,” ZDNET, February 5, 2020. View in Article
The article was first published here.
Photo by Google DeepMind.