Clean, governed data is table stakes for enterprise AI, and knowledge is the power that will differentiate the success of AI.
In brief
- Making raw data suitable for use by AI reduces the cost of training, improves the accuracy of responses and makes models adaptable for broader use cases.
- Organisations must expand the definition of data to include knowledge assets, a higher-value form of data that is used for AI decisions and actions.
- Organisations should establish an AI strategy that includes the creation of AI ready data.
Among open-source tech players and those backed by venture capital, the race is on to build the most accurate artificial intelligence (AI) models and capture AI market share. These AI startups are spending billions of dollars in capital on computing power, people and data to train AI models and improve AI inference. However, the large capital expense required to train public models does not easily correlate to the development of private AI models that leverage enterprise data. Companies that are experimenting with AI are realising that the potential value achieved from these AI pilots is outweighed by the cost to train the AI model for a single use case.
Early adopters of enterprise AI are taking a different approach to reduce the cost of training and drive broad adoption and success with private models. Business and IT functions are focusing on building AI ready data that is used to build corporate knowledge, which is then used to form knowledge maps that enhance the effectiveness of responses and make training AI models easier. Knowledge maps ultimately reduce the cost of implementing enterprise AI and create an experience similar to ChatGPT using company data, which provides contextualised responses that build trust in AI.
Chapter 1: Making Data AI Ready and Relevant
Raw data must be given context, and it must connect to the wider enterprise’s knowledge for AI provide more value
When the term “AI ready data” is mentioned, many people associate it with attributes like managed, governed, quality and trusted data. These characteristics correctly describe raw data sets of transactional and master data that is used for enterprise reporting. However, for AI, such raw data lacks context and actionable insights and typically produces incorrect answers or hallucinations. To achieve better results, technology companies are experimenting with retrieval augmented generation (RAG), directed acyclic graphs, vector databases, document parsing, agents and more — all in an effort to direct AI to the raw data with the appropriate relevance for the use case. These technology approaches are being taken to reduce the time and resources required to train AI, yet the solution to the problem must include a business approach to creating AI ready data and providing AI with easily traversable business knowledge.
Private AI models that support the enterprise or business functions require knowledge that is contained within the organisation’s process maps, charters, business architectures, control frameworks, etc.
These business documents and the information within them provide much-needed context when connected with transactional and master data. When this raw business data can be easily connected, it becomes a knowledge asset that forms business knowledge.
AI ready data is information that is easily combined to form business knowledge. These knowledge assets are used to enhance enterprise AI models, improving AI inference. AI ready data is a higher-value form of data that is used for decisions and actions.
Many AI strategies do not consider how to create AI ready data, instead focusing on the pilots or proofs of concept (POCs) that experiment with technology and address individual use cases. These pilots are difficult to manage and scale beyond an individual use case and inevitably create challenges. Corporate AI strategies must expand the enterprise definition of data to include knowledge assets. A well-formed AI strategy includes the creation and management of knowledge that exists at the enterprise level as well as within each business function.
Enterprise Raw Data
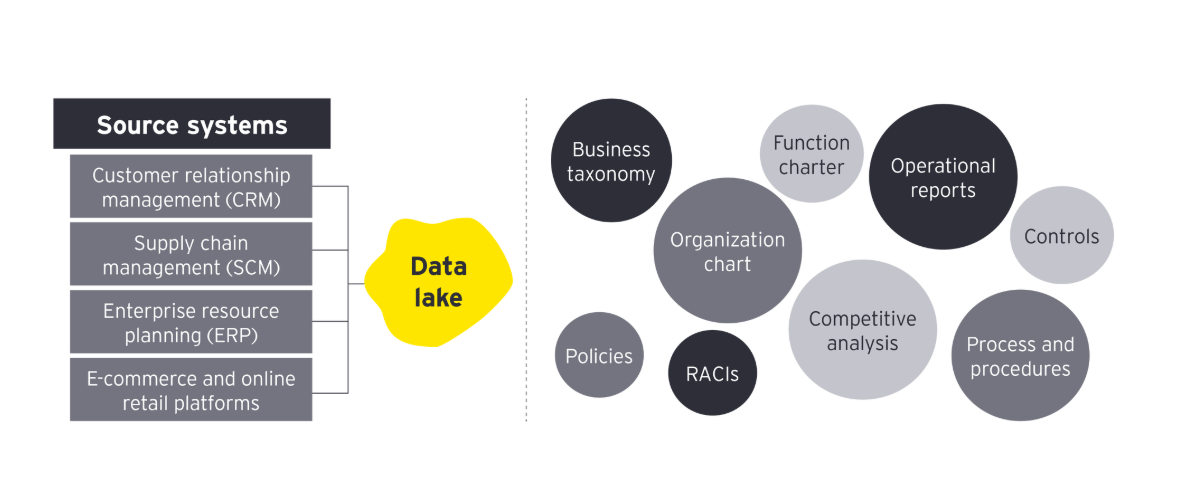
This chart illustrates the flow of data from various source systems into a data lake, which then feeds into different business functions.
1. Source systems: On the left side, there is a box labeled “Source systems” containing four rectangular blocks that list the following systems:
- Customer relationship management (CRM)
- Supply chain management (SCM)
- Enterprise resource planning (ERP)
- E-commerce and online retail platforms
2. Data lake: These source systems are connected by lines to a yellow blob in the center labeled “Data lake.”
3. Business functions: On the right side of the chart, there are several circles of varying sizes, each representing different business functions that utilize the data from the data lake. These circles are labeled as follows:
- Business taxonomy
- Function charter
- Operational reports
- Controls
- Organisation chart
- Competitive analysis
- Process and procedures
- RACIs
Chapter 2: The Importance of Knowledge Assets
AI ready daya makes it possible for organisations to develop enterprise- and function-specific knowledge assets that can help improve AI model’s accuract and reduce the cost of training
Imagine that a company hired a world-class industry expert. On day one, do they start making decisions about the corporate processes, or does the company invest in knowledge transfer to ensure the success of the resource investment? Such is the same for enterprise AI models: Companies need to capture and document the know-how to get the optimal accuracy for their use. To accelerate corporate knowledge, many companies have created enterprise ontologies, taxonomies and business glossaries to bring alignment across all business functions but never intended to use them to direct AI prompts and responses. Yet these are the frameworks and structures that are ideal for accelerating the accuracy and inference of enterprise AI models.
The enterprise ontology, taxonomy and business glossary, which together establish a shared vocabulary that aligns all knowledge assets, are the table stakes required for AI ready data.
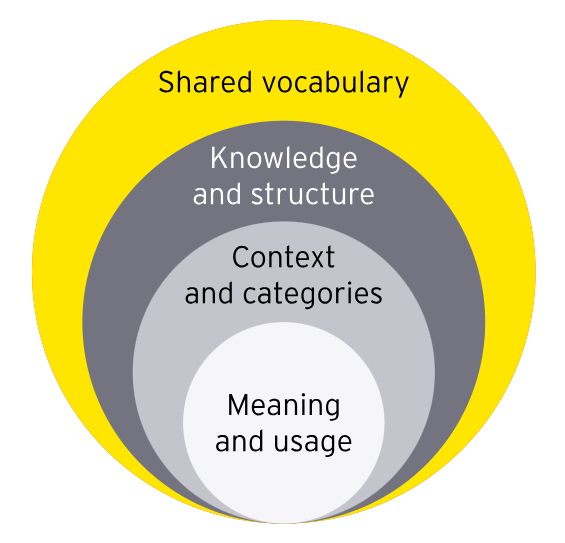
Business Glossary
A business glossary is a means to store information about data such as meaning, relationships to other usage and format.
There may be many different purpose-built business glossaries depending on audience and use
Taxonomy
A taxonomy is a way to organise data int categories and subcategories.
Taxonomies aim to address ambiguity, as the same term can have a different meanings.
Taxonomies include mechanisms for understanding context and making meaning precise.
Ontology
An ontology established a shared vocabulary that aligns the business concepts that exist.
They are structural frameworks for organising information as knowledge.
An ontology is intended to represent entities, ideas, and events, along with their properties and relations, according to a system of categories.
Additional enterprise knowledge assets include enterprise architecture documentation that unifies the business architecture with the data architecture and system architecture. Individually, these documents represent raw business data; but when combined, they become valuable enterprise knowledge assets.
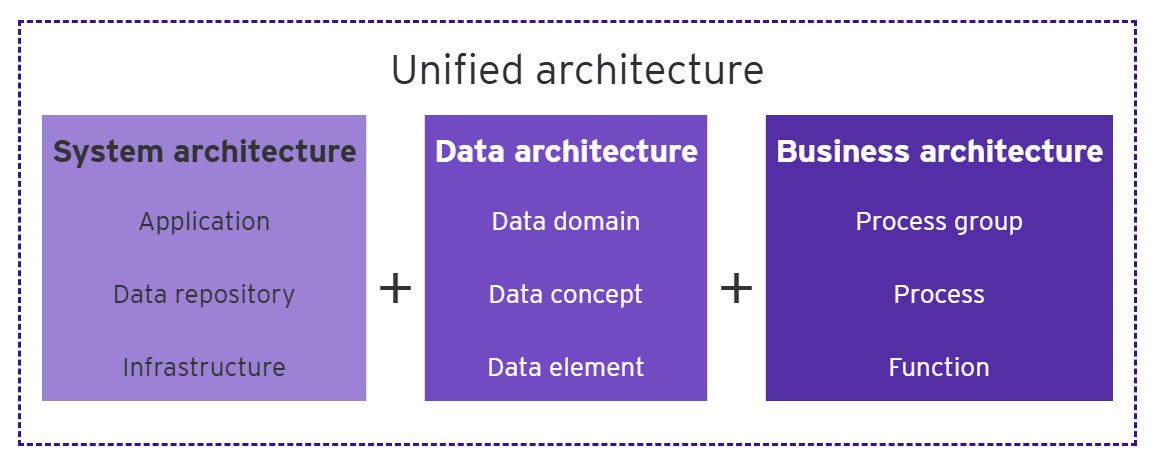
Function-specific knowledge assets are commonly anchored to a business process. Mapping various types of raw data — including applications, as well as information about the organisation’s risks, controls and people — to a business process provides valuable business knowledge that can easily enhance a function-specific AI model’s responses. Typically, it is not easy to connect this raw data together. Making the data suitable for use by AI will require function-specific initiatives. Once these knowledge assets are ready to connect, functions can create knowledge maps to help AI traverse the relationships between different pieces of information providing business context.
Business Function Knowledge
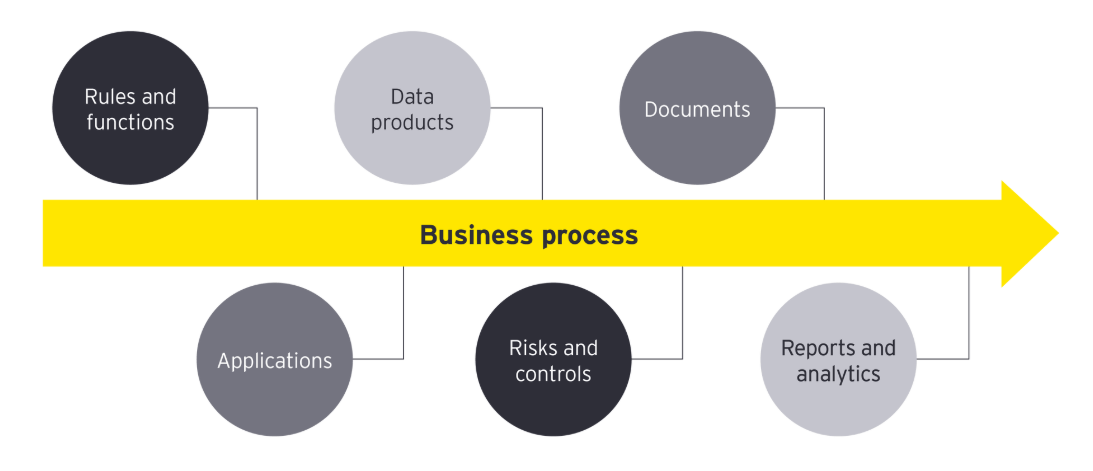
This chart visually represents various components that interact with a central “Business process.”
1. Central element: A horizontal arrow pointing to the right with the label “Business process”.
2. Connected elements: Surrounding the central arrow are six circular nodes, each connected to the arrow. These nodes represent different components that interact with the business process. They are labeled as follows:
- Top left: “Rules and functions”
- Top center: “Data products”
- Top right: “Documents”
- Bottom left: “Applications”
- Bottom center: “Risks and controls”
- Bottom right: “Reports and analytics”
By providing context from the retrieved data, the knowledge map improves the efficacy of AI when used to parse documents, build a RAG architecture or vector database, or create an agent. This context will help build confidence in the response as well as enhance the prompts to refine the results. Building a functional and enterprise AI model on knowledge assets greatly expands the number of use cases supported and makes achieving a high degree of AI inference easier.
Business functions typically have a wealth of documents that could be considered raw data. When a business function aligns the raw documents to the enterprise ontology and taxonomy, they become knowledge assets that can be connected to enterprise models.
Companies that haven’t included the creation of knowledge assets as part of their AI strategy require coordinated and focused efforts to transform existing structured and unstructured raw data into AI ready data. A comprehensive strategy will also include the creation of tacit knowledge. This knowledge is not always documented or maintained and resides in the minds of the most experienced employees. Filling gaps between knowledge assets with tacit knowledge will improve AI inference and reduce the cost of training. The current focus on AI use cases must also prioritise knowledge assets. Business functions can start by prioritising the creation of knowledge assets and tacit knowledge that support their AI use case; the functions can continue to add knowledge assets with each use case.
AI Ready Data
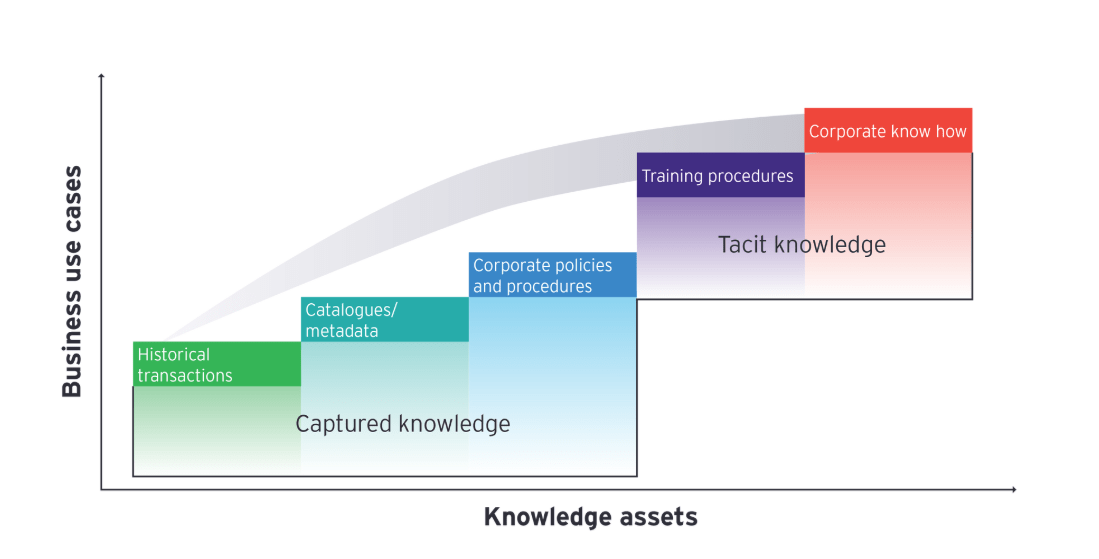
A line chart that shows the relationship between business use cases and knowledge assets.
1. Captured knowledge includes:
- Historical transactions
- Catalogues/metadata
- Corporate policies and procedures
2. Tacit knowledge includes:
- Training procedures
- Corporate know how
Business transformation initiatives are also a great opportunity to initiate the creation of knowledge assets. Transformations, which involve fundamental changes to how a business or organisation is run, produce numerous deliverables across a wide range of stakeholders —deliverables that can then be turned into knowledge assets for AI.
To complicate matters, most business applications that are used to create these deliverables, like process modeling tools and Word, PowerPoint and Excel documents, are not always configured to create knowledge assets. IT teams will need to partner with business stakeholders to form an approach so that the raw data can be transformed into a knowledge asset and become AI ready data.
Enterprise AI Knowledge
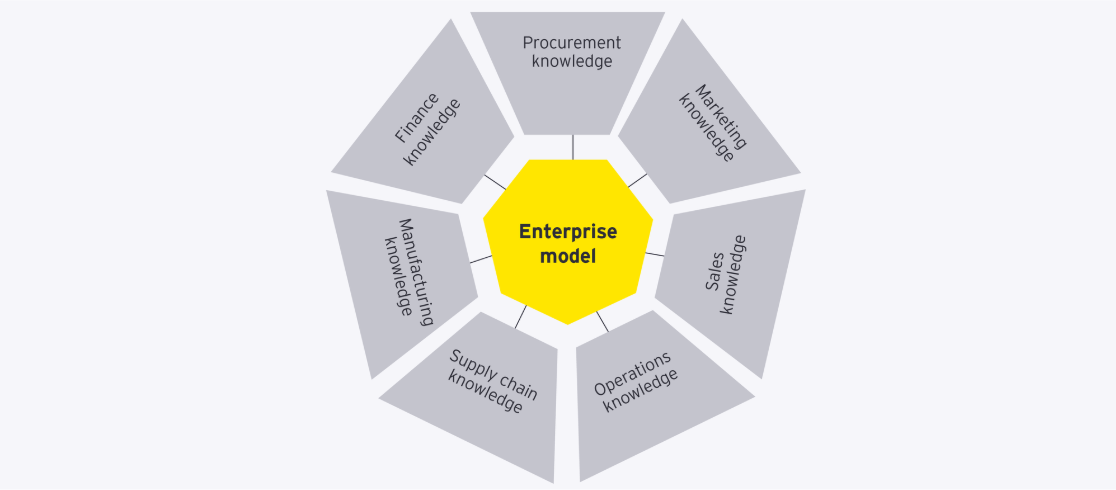
This chart is a visual representation of an enterprise model, depicted as a central hexagon labeled “Enterprise model.” Surrounding this central hexagon are seven pentagons, each representing a different area of knowledge essential to the enterprise model. These areas are:
- Procurement knowledge
- Marketing knowledge
- Sales knowledge
- Operations knowledge
- Supply chain knowledge
- Manufacturing knowledge
- Finance knowledge
Chapter 3: Strengthening the Organisation’s Focus on AI Ready Data
There are several leading practices companies can adopt to make the training of enterprise AI models less costly and easier.
Employees are generating volumes of raw data in the form of business documents, reports, transactions, process maps, etc. To reduce the capital expense required to train enterprise AI, support many use cases, improve the accuracy of prompts and achieve adoption, consider the following initiatives:
- Establish an enterprise AI knowledge management approach as part of the AI strategy. AI knowledge management will ensure that every program and project is producing AI ready knowledge assets. Teams across functions work at varying paces. Having an AI knowledge management framework will allow them to work independently, document the tacit knowledge and accelerate the creation of knowledge maps.
- Establish an enterprise business ontology, taxonomy and business glossary that will align the enterprise to a common language. This language will unify the business functions in the creation of knowledge assets.
- Treat all corporate information as a digital asset by employing the concepts of agile product management in the creation of data, reports and documents. This will ensure that owners of the digital assets are focused on addressing stakeholder needs including AI. Many companies have adapted this leading practice to data and reports, but it can also be applied to business documents. Every digital asset should be managed as a product and built as a knowledge asset.
- Establish a succinct number of cross-functional foundational data products that support the enterprise. Directing AI to foundational data products that have business context will remove the complexity of AI having to traverse the large number of tables and views that exist in a data lake.
- Focus on the metadata for all enterprise information. Metadata is a key component in creating knowledge and connecting information assets. Many companies have processes to capture business, technical and operation metadata for their transactional and master data. These same concepts can be applied to all business documents.
Summary
The excitement created by open-source AI models is prompting companies to replicate this success using internal data. Companies do not have the luxury of spending millions to build and train enterprise AI without measurable business value. Through focused efforts to establish knowledge assets, companies can quickly release AI models that support multiple use cases and eliminate ad hoc reports. With easy access to all the documented knowledge, executives can imagine the opportunities that lie ahead.
The article was first published by EY.
Photo by Mika Baumeister on Unsplash.